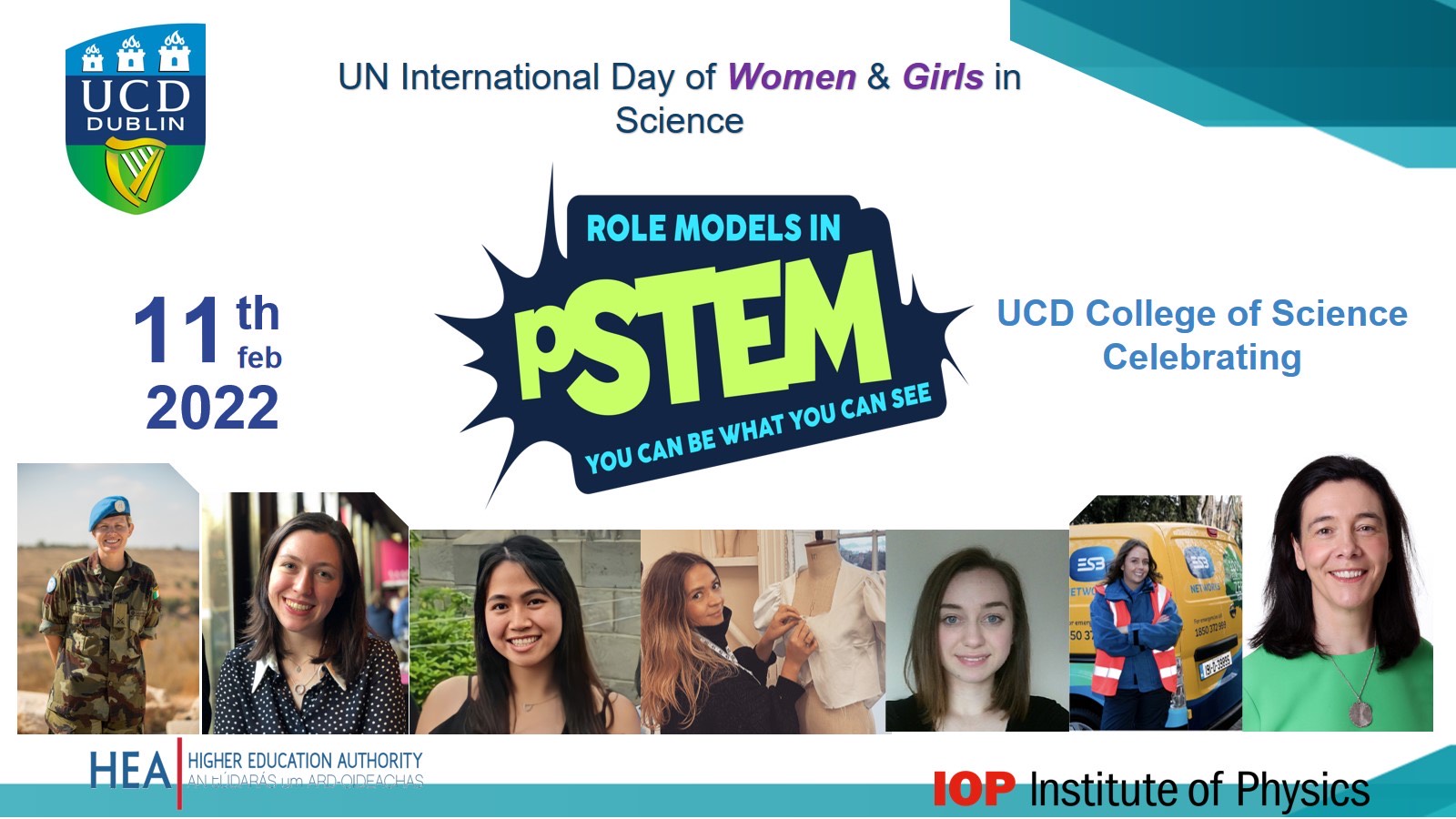
We are proud to celebrate International Day of Women and Girls in Science in UCD today.
UCD is marking International Day of Women and Girls in Science by launching a series of videos to encourage more girls to consider a career in pSTEM. The video series, entitled “Role Models in pSTEM: You Can Be What You Can See”, showcases ten female role models from across Ireland who have studied physics, maths, engineering, or computer science. Three of the videos were revealed at an event in UCD today to mark International Day of Women and Girls in Science.
Commissioned by UCD’s School of Mathematics and Statistics, and School of Computer Science, the full suite of videos will launch in May 2022. The videos will be accompanied by an educational resource for teachers which can be used in schools across Ireland – with a particular focus on DEIS schools – to encourage students to identify their own local role models, while highlighting the varied and exciting career opportunities open to young women in pSTEM.
Leading the project are Dr. Aoibhinn Nà Shúilleabháin, Assistant Professor at the School of Mathematics and Statistics, and Dr. Catherine Mooney, Associate Professor in the School of Computer Science at University College Dublin.
We (Lan Wei, Yuhan Du, Shamima Nasrin Runa) are proud to volunteer and participate in the event to hear the inspiring talks by the female role models.
You can find the videos on our YouTube channel: https://www.youtube.com/channel/UCYrh8Eh848_Ljzfx5BXiE9A
Our temporary webpage (to be updated for the full launch) is here: https://www.ucd.ie/mathstat/rolemodelsinpstem/
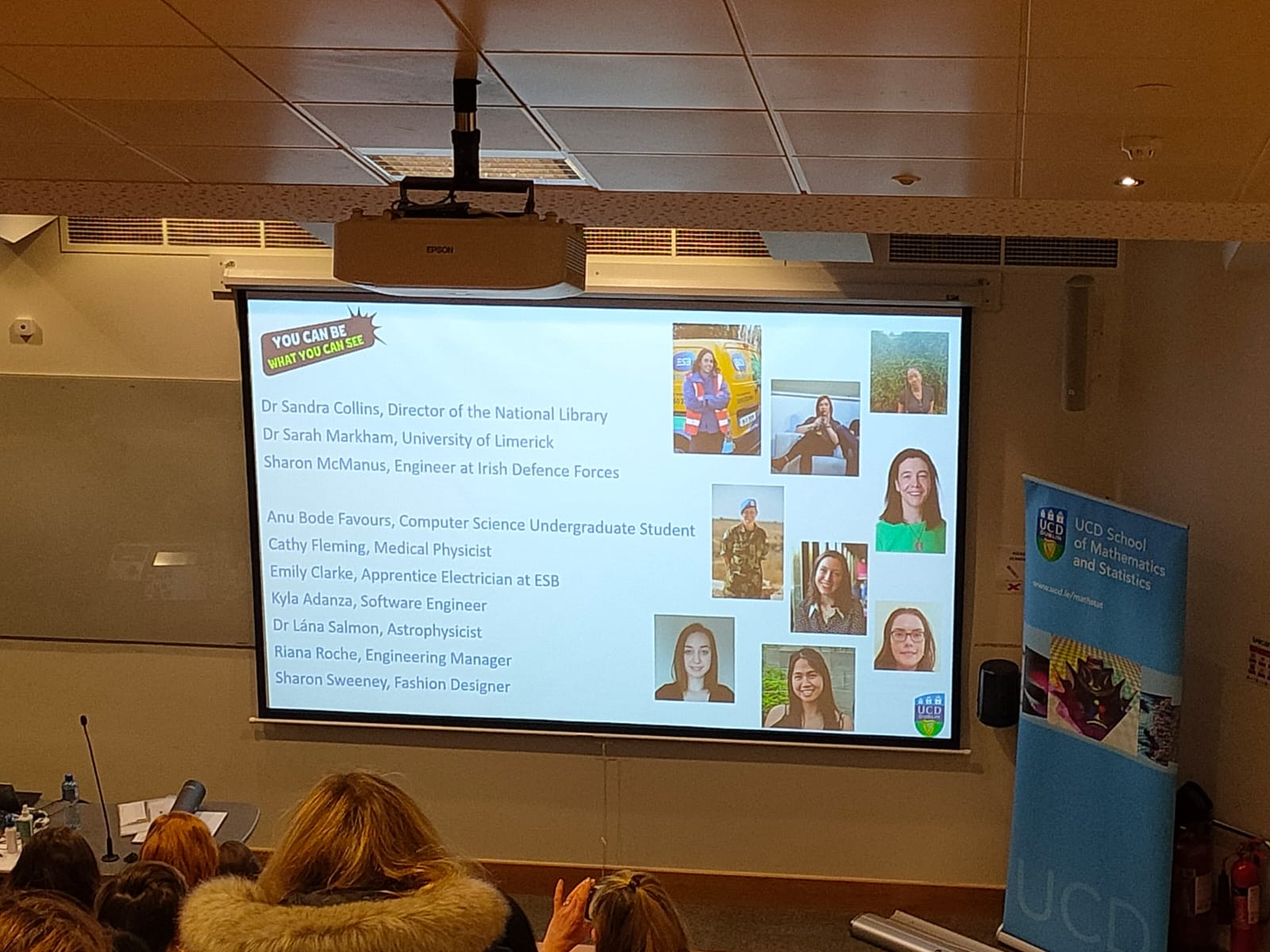
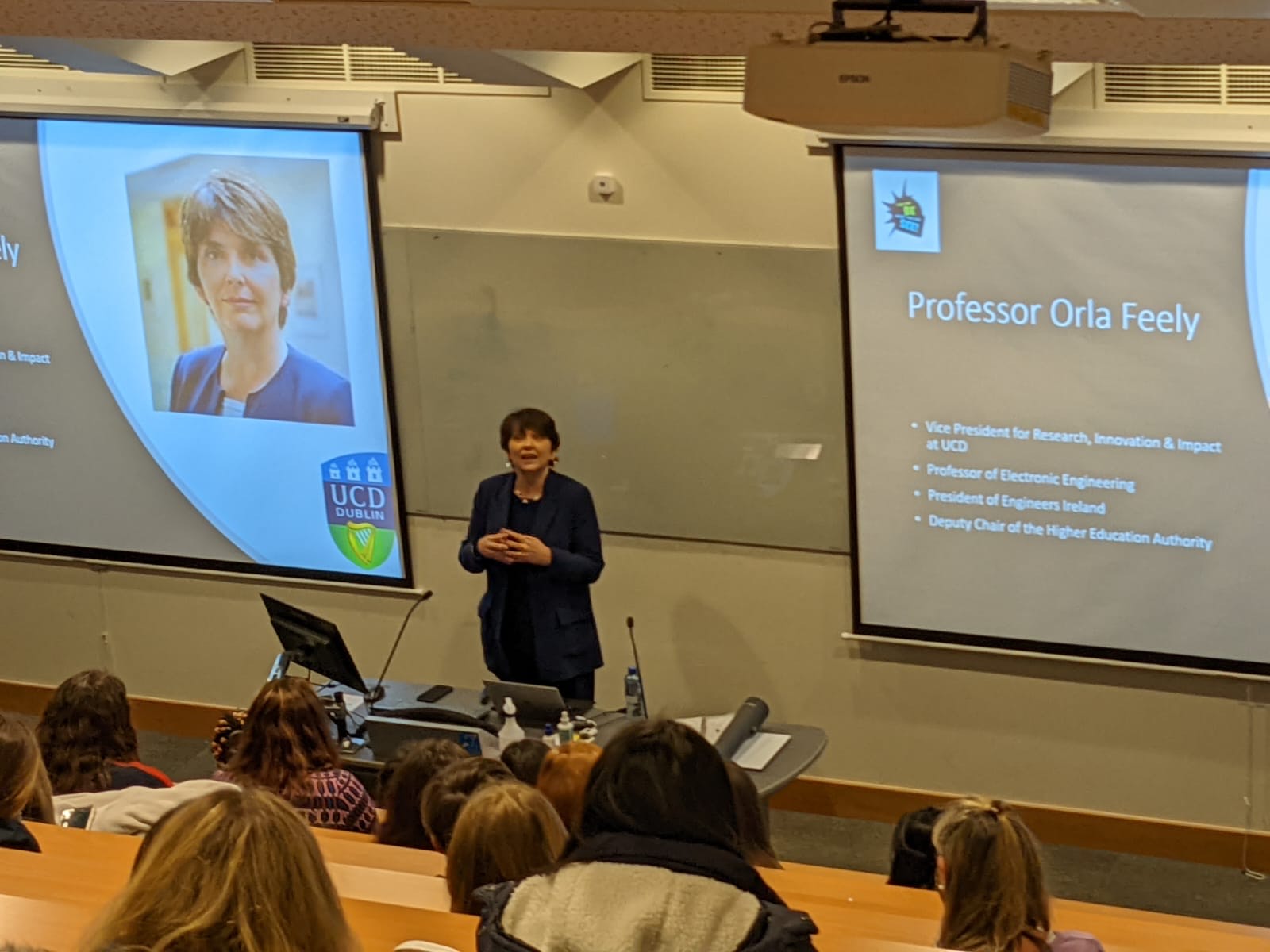
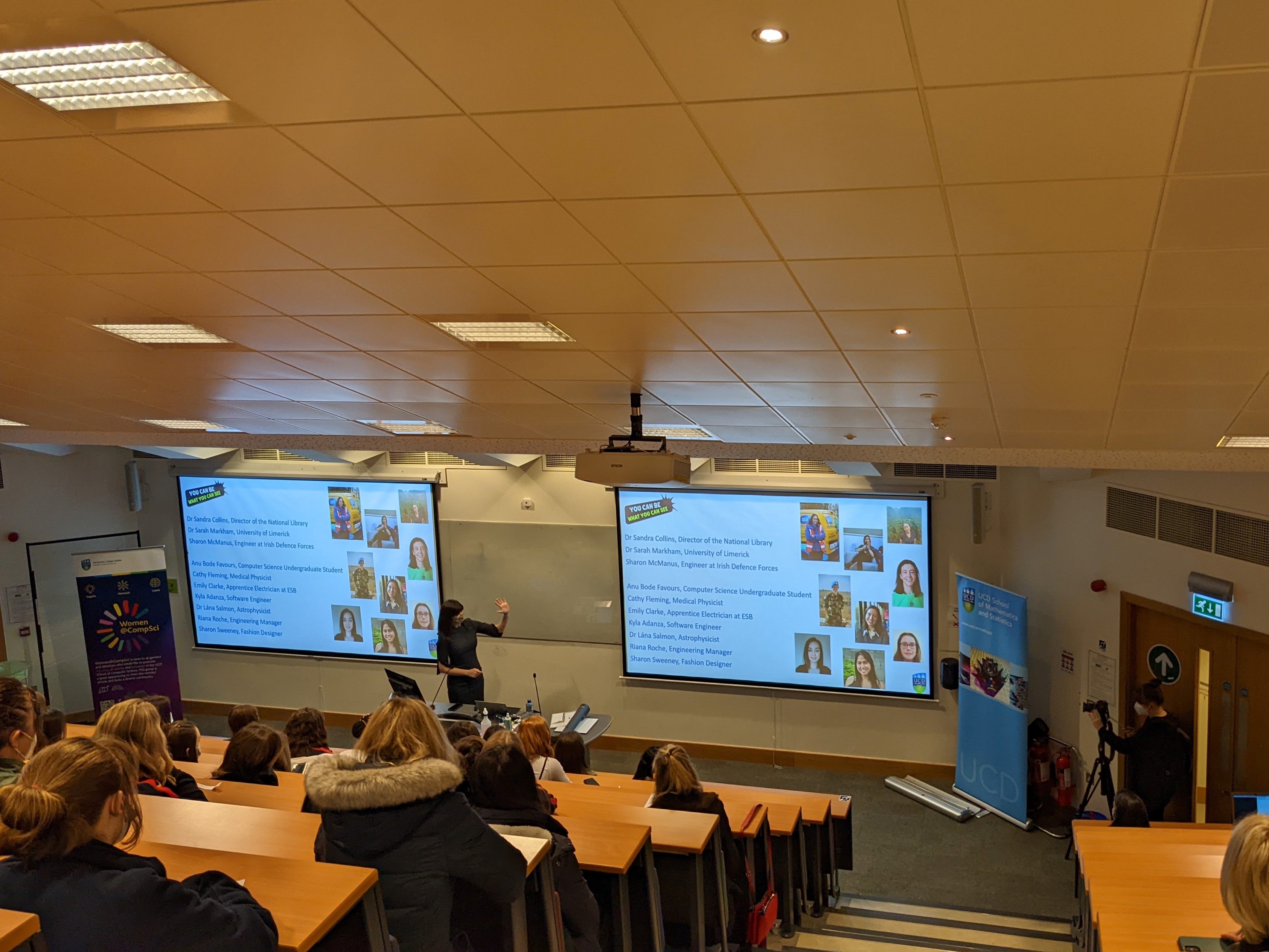
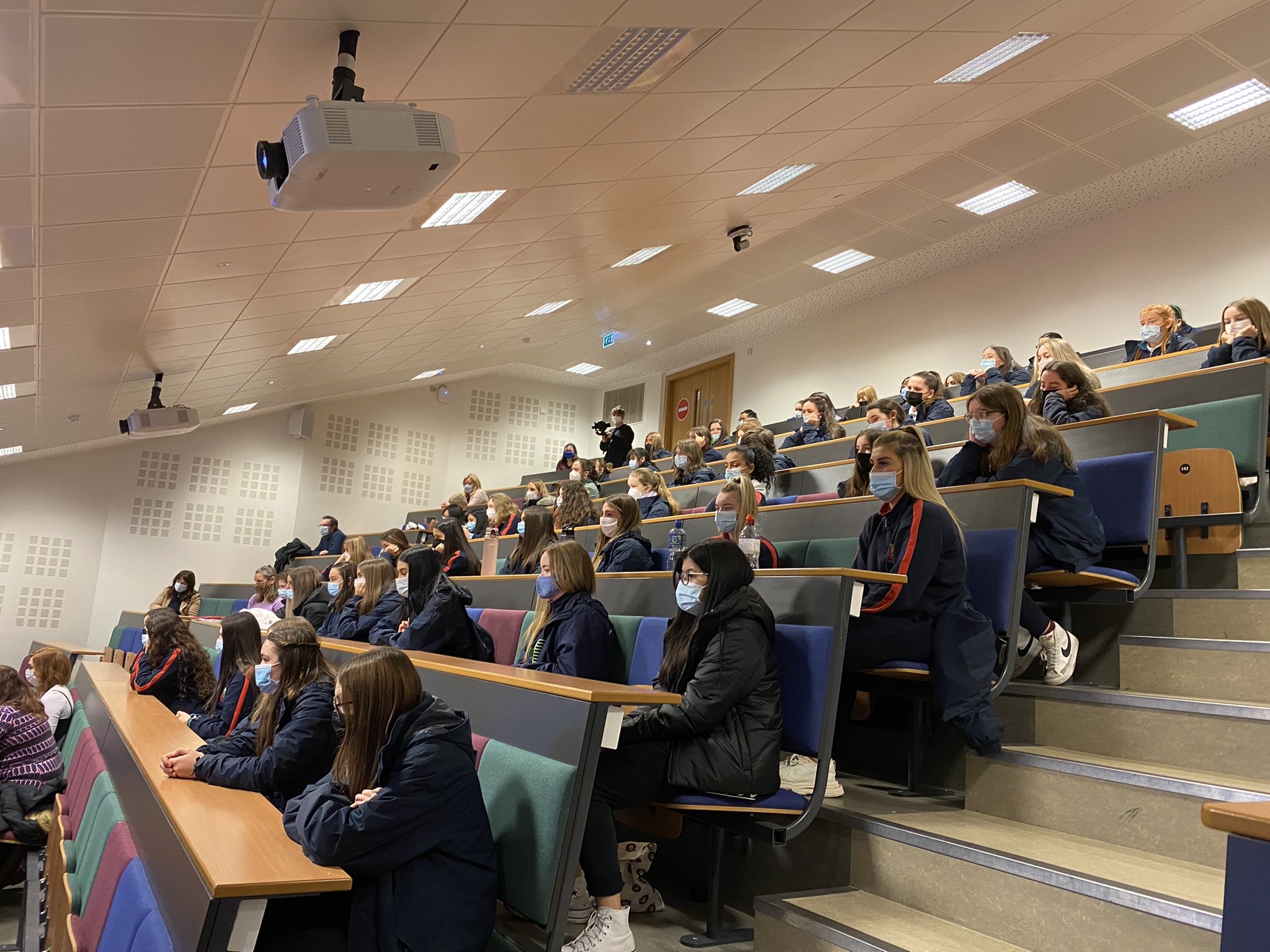
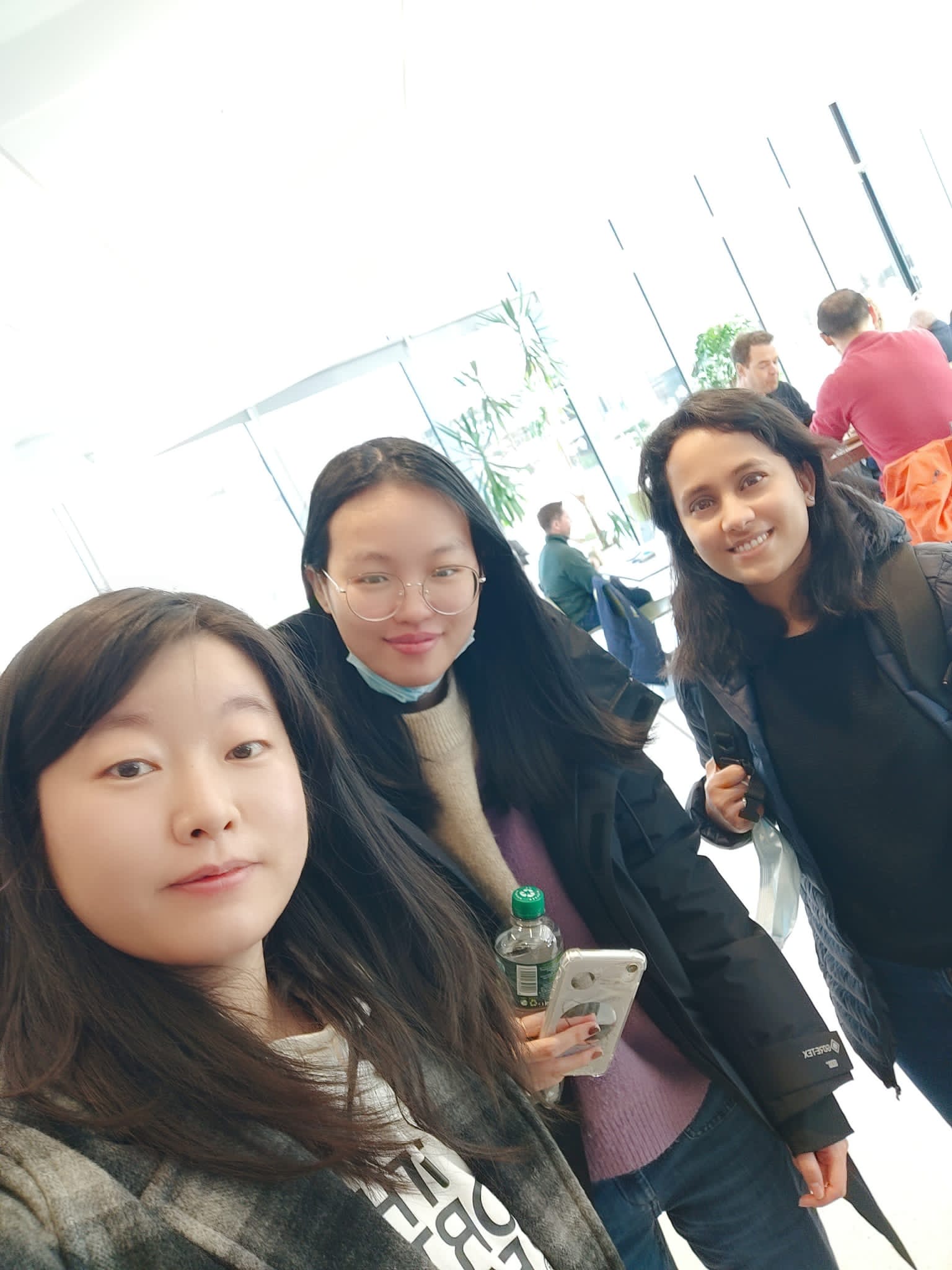