A refreshing morning together with coffee. It was lovely to meet you all in person. ☺️
“Science Day in UCD”
Celebrating Science Day in UCD
Today there were some student societies who demonstrated their extraordinary scientific experimental discoveries and also showed some very interesting projects. While physics societies have shown their formula for making liquid ice cream using physics equations, the School of Earth Science societies have been presented with a few rare collections of stone, glass, and fossils. Women+ in STEM Society put music in STEM. They showed off their banana piano tests there and they also added another test called Glow-in-the-Dark Jar. There were couple of societies who also have participated this demonstration table to successfully celebrate Science day in UCD.
You Can Be What You Can See: Role Models in pSTEM
We are proud to celebrate International Day of Women and Girls in Science in UCD today.
UCD is marking International Day of Women and Girls in Science by launching a series of videos to encourage more girls to consider a career in pSTEM. The video series, entitled “Role Models in pSTEM: You Can Be What You Can See”, showcases ten female role models from across Ireland who have studied physics, maths, engineering, or computer science. Three of the videos were revealed at an event in UCD today to mark International Day of Women and Girls in Science.
Commissioned by UCD’s School of Mathematics and Statistics, and School of Computer Science, the full suite of videos will launch in May 2022. The videos will be accompanied by an educational resource for teachers which can be used in schools across Ireland – with a particular focus on DEIS schools – to encourage students to identify their own local role models, while highlighting the varied and exciting career opportunities open to young women in pSTEM.
Leading the project are Dr. Aoibhinn Ní Shúilleabháin, Assistant Professor at the School of Mathematics and Statistics, and Dr. Catherine Mooney, Associate Professor in the School of Computer Science at University College Dublin.
We (Lan Wei, Yuhan Du, Shamima Nasrin Runa) are proud to volunteer and participate in the event to hear the inspiring talks by the female role models.
You can find the videos on our YouTube channel: https://www.youtube.com/channel/UCYrh8Eh848_Ljzfx5BXiE9A
Our temporary webpage (to be updated for the full launch) is here: https://www.ucd.ie/mathstat/rolemodelsinpstem/
XAI for Prediction of Gestational Diabetes
Our paper “An explainable machine learning-based clinical decision support system for prediction of gestational diabetes mellitus” is published in Scientific Reports today.
Gestational Diabetes Mellitus (GDM), a common pregnancy complication associated with many maternal and neonatal consequences, is increased in mothers with overweight and obesity. Interventions initiated early in pregnancy can reduce the rate of GDM in these women, however, untargeted interventions can be costly and time-consuming. We have developed an explainable machine learning-based clinical decision support system (CDSS) to identify at-risk women in need of targeted pregnancy intervention. Maternal characteristics and blood biomarkers at baseline from the PEARS study were used. After appropriate data preparation, synthetic minority oversampling technique and feature selection, five machine learning algorithms were applied with five-fold cross-validated grid search optimising the balanced accuracy. Our models were explained with Shapley additive explanations to increase the trustworthiness and acceptability of the system. We developed multiple models for different use cases: theoretical (AUC-PR 0.485, AUC-ROC 0.792), GDM screening during a normal antenatal visit (AUC-PR 0.208, AUC-ROC 0.659), and remote GDM risk assessment (AUC-PR 0.199, AUC-ROC 0.656). Our models have been implemented as a web server that is publicly available for academic use. Our explainable CDSS demonstrates the potential to assist clinicians in screening at risk patients who may benefit from early pregnancy GDM prevention strategies.
Check it out at: https://rdcu.be/cFqM3
–Yuhan
The Irish Times featuring LiSDA
Anna Markella Antoniadi was interviewed for the “Research Lives” column in the Irish Times. She discussed her work on Amyotrophic Lateral Sclerosis and her life as a researcher; academic interests and hobbies, moving to Ireland, completing her PhD.
You can find her interview here.
Lan Wei’s PhD Viva
Congratulations to Lan Wei who successfully passed her PhD Viva this morning.
Lan’s thesis title is “Automatic Detection and Characterization of Seizures and Sleep Spindles in Electroencephalograms Using Machine Learning”.
Many thanks to her external examiner, Prof. Lijuan Duan, and internal examiner, Dr Ruihai Dong. Many thanks also to Assoc. Prof. Brian Mac Namee for arranging and chairing the Viva via zoom, and to Lan’s co-supervisor, Prof. Madeleine Lowery, for her support and guidance of Lan over the last four years.Many thanks to Lan’s Research Studies Panel (Prof. Madeleine Lowery, Assoc. Prof. Gianluca Pollastri, and Dr Andrew Hines) for their advice during the past four years.
Finally, a big thank you to our collaborators, Soraia Ventura, Dr Gareth Morris, Prof. David C. Henshall, Dr Sean Mathieson, Prof. Geraldine B., Mary Anne Ryan, Halima Boutouil, Boylan Rogerio Gerbatin, Dr Cristina R Reschke, Dr Omar Mamad and Dr Mona Heiland for their assistance, patience, attention and all the advice throughout Lan’s PhD.
–Lan
LiSDA@Epi-AI
Delighted our paper ‘Detection of spontaneous seizures in EEGs in multiple experimental mouse models of epilepsy‘ has been published in the Journal of Neural Engineering.
The Epi-AI webserver is also available online.
Epi-AI supports basic scientists to analyse EEG datasets by predicting if events are seizure events or not. Epi-AI supports single-channel EEG recordings in EDF, CSV, and PICKLE formats. If you upload an EEG in EDF format please choose which channel you want to analyse. If you upload a PICKLE or CSV file please choose which channel you want to analyse, the sampling frequency and the start time of your EEG recordings. Information about each detected seizure e.g. the number of seizures, start time, end time, duration, amplitude and corresponding spectrogram, will be available for download allowing for further analysis.
–Lan
LiSDA@Spindle-AI
Delighted our paper ‘Spindle-AI: Sleep spindle number and duration estimation in infant EEG‘ has been published in IEEE Transactions on Biomedical Engineering.
The Spindle-AI webserver is also available online.
Spindle-AI allows the user to choose the sampling frequency of their data and submit a CSV file that contains a single-channel EEG signal. Spindle-AI will predict if the events are sleep spindle events or non-sleep spindle events. Spindle-AI then returns the start time, end time and the total number of the detected sleep spindle events.
–Lan
LiSDA @ vGHC21
I (Yuhan Du) attended the Grace Hopper Celebration 2021 on Sep 27 – Oct 1 and presented my poster “Early Prediction of Macrosomia Using Machine Learning”, coauthored with Dr. Catherine Mooney. The poster described our research on predicting fetal macrosomia, which refers to infants born with excessive birth weight. In this study, we developed machine learning models to predict macrosomia in the early second trimester in secundigravid women who had a macrosomic birth history with a novel inclusion of usability. Our work has the potential to assist pregnancy care in a clinical setting.
You can find our poster at: https://vghc21-anitab.ipostersessions.com/Default.aspx?s=FF-75-6E-65-72-A8-15-7C-1A-08-33-9E-11-38-C7-A0
–Yuhan Du
Anna Markella Antoniadi’s PhD Viva
Congratulations to Anna Markella Antoniadi who successfully passed her PhD viva this afternoon.
Anna’s thesis title is “The Application of Explainable Artificial Intelligence in Modelling Quality of Life and Caregiver Burden in Amyotrophic Lateral Sclerosis to Inform Clinical Decision-Making”.
Many thanks to her examiners, Prof. Pierangelo Veltri, University of Catanzaro, and internal examiner Dr Mark Matthews. Many thanks also to Associate Prof. Gianluca Pollastri for arranging and chairing the viva via zoom, and to Anna’s Research Studies Panel (Prof. Pádraig Cunningham and Dr Marguerite Barry) for their support and guidance of Anna over the last four years. Finally, a big thank you to our collaborators, Prof. Orla Hardiman, Miriam Galvin and Mark Heverin in Trinity College Dublin for closely working with Anna throughout her PhD.
Staying Connected…
…in Ireland, Greece, China, Colombia, Mexico
LiSDA @ ACM BCB 2021
I (Yuhan Du) attended the 12th ACM Conference on Bioinformatics, Computational Biology, and Health Informatics (ACM BCB) https://acm-bcb.org/ that was held online on Aug 1-4, 2021. I presented my poster entitled “Explaining Large-for-Gestational-Age Births: A Random Forest Classifier with a Novel Local Interpretation Method”, coauthored with Dr. Anthony R Rafferty, Prof. Fionnuala M McAuliffe and Dr. Catherine Mooney. In the poster, the authors proposed a novel local interpretation method for a random forest classifier based on feature occurrence frequency in trees that give the same prediction as the random forest classifier. The method shows promising results when applied to a random forest classifier for large-for-gestational-age births.
– Yuhan
What’s it like to be an MSCA fellow? Hear from Claudia Mazo
What’s it like to be a Marie-Sklodowska Curie Actions Fellow?
Check out these testimonials from our Postdoc Claudia Mazo
https://audiovisual.ec.europa.eu/en/video/I-201997?lg=ES%2FEN
She would really recommend any early-career research to apply for this great opportunity. As she said: “This fellowship has allowed me to have the benefits of academia and industry together….. to broaden my current knowledge and develop technical, industrial, and research skills”. Having the opportunity to collaborate with different experts in her field from around the world also has made her much more competitive.
Global Motor Neurone Disease (MND) Awareness day 21/06/2021
Today is Global Motor Neurone Disease (MND) Awareness day. You can read about our recent work on MND/ALS here: https://www.futureneurocentre.ie/news/als-caregivers-quality-of-life-could-be-predicted-by-computer-systems/
You can find more about #MND in Ireland or donate at imnda.ie.
The main points of our paper on the “Development of an explainable clinical decision support system for the prediction of patient quality of life in amyotrophic lateral sclerosis” explained!
[pdf-embedder url=”https://lisda.ucd.ie/wp-content/uploads/2021/06/publication.pdf”]
Our Work on Caregiver Burden in “ALS News Today”
An article on our BMJ Open paper “Prediction of caregiver burden in amyotrophic lateral sclerosis: a machine learning approach using random forests applied to a cohort study” was published on “ALS News Today“!
LiSDA @ ACM SAC 2021
Anna Markella Antoniadi attended the ACM SAC 2021 virtual conference and presented our work on the “Development of an Explainable Clinical Decision Support System for the Prediction of Patient Quality of Life in Amyotrophic Lateral Sclerosis” at the Health Informatics Track of the Conference. This work is a collaboration with Miriam Galvin, Mark Heverin and Orla Hardiman and it is a work towards the development of the first Clinical Decision Support System to automatically predict the Quality of Life (QoL) of people with Amyotrophic Lateral Sclerosis, easily and quickly, in order for clinicians to decide on the necessary supports. We found that the predictors of patient QoL are their age at disease onset, orthopnoea score, as well as their primary caregiver’s employment status before the disease onset. More details can be found in our paper in the proceedings.
[pdf-embedder url=”https://lisda.ucd.ie/wp-content/uploads/2021/03/ACM-SAC-2021-FIRST-SLIDE.pdf” title=”ACM-SAC 2021 FIRST SLIDE”]
LiSDA @ AMIA Informatics Summit 2021
I (Yuhan Du) am proud to present my poster entitled “Prediction of Gestational Diabetes Mellitus in Overweight and Obese Caucasian Women using Machine Learning” coauthored by Professor Fionnuala M McAuliffe and Dr Catherine Mooney at AMIA Informatics Summit 2021 on March 23, 2021.
The poster presents our initial results on predicting gestational diabetes mellitus (GDM) in overweight and obese Caucasian pregnant women. GDM is a common pregnancy complication associated with many maternal and neonatal consequences, and it is increased in risk by overweight and obesity. We applied machine learning to develop prediction models for GDM in overweight and obese Caucasian women to identify at-risk women in need of intervention early in pregnancy. This work was based on maternal characteristics and blood biomarkers at baseline from the PEARS study. After appropriate data preparation and feature selection, five machine learning algorithms were applied with synthetic minority oversampling technique and five-fold cross validation repeated five times optimising the area under precision-recall curve. Our results showed that a support vector machine with polynomial kernel outperformed other algorithms, identifying 44% and 67% of GDM women in early pregnancy at 5% and 10% false positive rate respectively. This work demonstrates the potential of applying machine learning to the prediction of GDM in a clinical setting.
[pdf-embedder url=”https://lisda.ucd.ie/wp-content/uploads/2021/03/PS01_Du.pdf”]
–Yuhan
LiSDA @ SIGCSE TS 2021
We (Brett Becker and Catherine Mooney) are just “back” from the 52nd ACM Technical Symposium on Computer Science Education (SIGCSE TS) that was held virtually on March 13-20, 2021 (https://sigcse2021.sigcse.org/).
We presented a paper “Investigating the Impact of the COVID-19 Pandemic on Computing Students’ Sense of Belonging” which won a best paper award (https://dl.acm.org/doi/abs/10.1145/3408877.3432407).
Student sense of belonging has been shown to be associated with many attributes such as motivation and persistence. However, sense of belonging can show variations according to factors such as race and gender. In this study, we examined the impact that the COVID-19 pandemic has had on our students’ sense of belonging .
We found that:
COVID-19 had a larger impact on the sense of belonging of all students in the space of a few months than we otherwise observed over the two prior years.
Men and women who do not identify as being part of any minority appear to have had similar downward shifts in their senses of belonging. Although in our results only men showed a statistically significant decrease, the lack of a significant difference in women is likely due to sample size.
Men who do not identify as being part of any minority saw the largest statistically significant drop in belongingness post-COVID-19.
Women who do identify as being part of a minority had a large mean increase in sense of belonging post-COVID-19 although this was not statistically significant. Again, sample size may be a factor.
This study has provided us with important insight into how a shift to online learning affected the belongingness of our students. At this point we can only conclude that these effects are real, complex, and affect individual students and groups differently – some negatively. The mechanisms of these effects are not yet understood. Solid evidence of causation is likely to take time to establish. If others have observed similar trends at different institutions, working together would likely help us understand these issues better.
— Catherine Mooney
LiSDA@IEEE-SPMB 2020 Special session
Lan and Catherine attended the 2020 IEEE Signal Processing in Medicine and Biology Symposium (IEEE-SPMB 2020), the conference of IEEE SPMB held virtually on December 5, 2020.
Lan presented a full paper co-authored by Catherine, titled “Epileptic Seizure Detection in Clinical EEGs Using an XGboost-based Method”. Epilepsy is one of the most common serious disorders of the brain, affecting about 50 million people worldwide. Electroencephalography (EEG) is an electrophysiological monitoring method which is used to measure tiny electrical changes of the brain, and it is frequently used to diagnose epilepsy. However, the visual annotation of EEG traces is time-consuming and typically requires experienced experts. Therefore, automatic seizure detection can help to reduce the time required to annotate EEGs. Automatic detection of seizures in clinical EEGs has been limited-to date.
[pdf-embedder url=”https://lisda.ucd.ie/wp-content/uploads/2020/12/TUH_ppt.pdf”]
In this study, we present an XGBoost-based method to detect seizures in EEGs from the TUH-EEG Corpus. 4,597 EEG files were used to train the method, 1,013 EEGs were used as a validation set, and 1,026 EEG files were used to test the method. Sixty-four features were selected as the input to the training set, and Synthetic Minority Over-sampling Technique was used to balance the dataset. Our XGBoost-based method achieved sensitivity and false alarm/24 hours of 20.00\% and 15.59, respectively, in the test set. The proposed XGBoost-based method has the potential to help researchers automatically analyse seizures in clinical EEG recordings.
— Lan
LiSDA@IEEE-SPMB 2020 Poster session
Halima Boutouil presented a poster co-authored by L. Wei, R. Gerbatin, O. Mamad, H. Boutouil, C. Reschke, M. Lowery, D. Henshall, G. Morris and C. Mooney, titled “ XGboost-based Method for Seizure Detection in Mouse Models of Epilepsy ” at the 2020 IEEE Signal Processing in Medicine and Biology Symposium (IEEE-SPMB 2020), the conference of IEEE SPMB held virtually on December 5, 2020.
[pdf-embedder url=”https://lisda.ucd.ie/wp-content/uploads/2020/12/SPMB_poster.pdf”]
Epilepsy is a chronic neurological disease which affects over 50 million people worldwide. EEG monitoring in rodent disease models of epilepsy is critical in the understanding of disease mechanisms and drug development. However, the visual annotation of EEG traces is time-consuming, typically requires experienced experts and is subject to low inter-observer reproducibility. Therefore, automated seizure detection has been proposed recently to help to reduce the time required to annotate EEGs and improve reproducibility. Research on the automatic detection of seizures in mouse EEGs has been limited to date. In this study, we present a TKEO-based and an XGBoost-based method to detect seizures in EEGs from intra-amygdala kainic acid (IAKA) and Dravet syndrome (DS) mouse models of epilepsy. The TKEO-based method mimics how experts typically detect seizures in this type of mouse model of epilepsy and demonstrated 74.4% sensitivity and 97.5% specificity in the IAKA test set; and 25.0% sensitivity and 98.0% specificity in the DS test set. For the XGBoost-based method, one IAKA mouse and one DS mouse were used to train the method. 19 features were selected as the input and Synthetic Minority Over-sampling Technique (SMOTE) was used to balance the dataset. The sensitivity and specificity of the XGBoost-based method were 86.6% and 93.3% in the IAKA test set, and 98.5% and 98.8% in the DS test set. The proposed XGBoost-based method generalized well on two mouse models of epilepsy, and has the potential to assist researchers in the automated analysis of seizures in single-channel mouse EEG.
— Lan
LiSDA @ IEEE ICHI 2020
Anna Markella Antoniadi attended the online conference between 30 Nov and 2 Dec 2020. She presented a poster titled “Identifying Features That Are Predictive of Quality of Life in People With Amyotrophic Lateral Sclerosis”, co-authored by Miriam Galvin, Mark Heverin, Orla Hardiman and Catherine Mooney. A great variety of attendees, talks and workshops made this online conference a lovely experience nonetheless.
[pdf-embedder url=”https://lisda.ucd.ie/wp-content/uploads/2020/12/ICHI-2020-poster.pdf” title=”ICHI 2020 poster”]
LiSDA @ ACM-BCB 2020
Yuhan, Anna and Catherine attended the 11th ACM Conference on Bioinformatics, Computational Biology and Health Informatics (ACM-BCB 2020), the flagship conference of ACM SIGBio held virtually between Sep 21 and 24, 2020.
Yuhan presented a poster co-authored by John Mehegan, Fionnuala McAuliffe and Catherine Mooney, titled “Prediction of Large for Gestational Infants in Overweight and Obese Women at Approximately 20 Gestational Weeks”. Large for gestational age (LGA) birth is an adverse pregnancy outcome associated with many maternal and perinatal complications, however, there isn’t any established rule to predict LGA in early pregnancy. This poster presents our preliminary work on addressing the prediction of LGA in the second trimester using machine learning. It shows the potential of applying machine learning techniques to assist clinical decision makings to prevent maternal and neonatal morbidity.
[pdf-embedder url=”https://lisda.ucd.ie/wp-content/uploads/2020/09/ACM-BCB-poster.pdf” title=”ACM-BCB poster”]
— Yuhan Du
Anna Markella Antoniadi presented a poster co-authored by Miriam Galvin, Mark Heverin, Orla Hardiman and Catherine Mooney on “Using Patient Information for the Prediction of Caregiver Burden in Amyotrophic Lateral Sclerosis”. Previous work had identified important predictors of caregiver burden using more information. Here the authors aimed to reduce the predictive features to patient information alone in order to explore the possibility of developing a more usable Clinical Decision Support System (CDSS) for the prediction of caregiver burden, following the General Data Protection Regulations (GDPR) data minimisation principle.
[pdf-embedder url=”https://lisda.ucd.ie/wp-content/uploads/2020/09/ACM-BCB-2020-poster.pdf” title=”ACM-BCB 2020 poster”]
-Anna Markella Antoniadi
LiSDA @ UKICER 2020
We (Brett Becker and Catherine Mooney) are just “back” from the 2nd Annual United Kingdom & Ireland Computing Education Research (UKICER) conference, held virtually on September 3 and 4.
We presented a paper entitled “Sense of Belonging: The Intersectionality of Self-Identified Minority Status and Gender in Undergraduate Computer Science Students”.
Creating inclusive learning environments for all students is of primary importance. Student sense of belonging is an important part of this. However, sense of belonging can show variations according to factors such as ethnicity and gender as well as influencing attributes such as motivation and persistence. We utilised a survey adapted from the “Math Sense of Belonging Scale” to examine the relationship between undergraduate computer science students’ sense of belonging, gender identity, and self-declared minority status. We observed a lower sense of belonging in students who identified as women who also self-identified as being part of a minority group. However, students who identified as women who did not identify as belonging to a minority had a sense of belonging comparable to those identifying as men. Our results provide insight that may help us improve the sense of belonging of our undergraduate students, particularly those identifying as women and as belonging to a minority in computer science. It has also brought to our attention that action needs to be taken to mitigate the potentially disproportionately negative consequences that COVID-19 may have on these students due to reduced opportunities for social interaction and the negative impact that this has on sense of belonging.
— Catherine Mooney
EMBS Virtual Academy 2020
The 42nd Annual International Conference of the IEEE Engineering in Medicine and Biology Society (EMBC) in conjunction with the 43rd Annual Conference of the Canadian Medical and Biological Engineering Society was offered virtually via the EMBS Learning Academy, July 20th – 24th, 2020.
Lan Wei presented a full paper entitled ‘Random Forest-based Algorithm for Sleep Spindle Detection in Infant EEG’. This paper co-authored by Dr. Catherine Mooney (UCD), Professor Madeleine Lowery (UCD), Soraia Ventura (UCC), Dr. Sean Mathieson(UCC), Professor Geraldine B. Boylan (UCC) as well as Mary Anne Ryan (UCC).
Sleep spindles are associated with normal brain development, memory consolidation and infant sleep-dependent brain plasticity and can be used by clinicians in the assessment of brain development in infants. Sleep spindles can be detected in EEG, however, identifying sleep spindles in EEG recordings manually is very time-consuming and typically requires highly trained experts. Research on the automatic detection of sleep spindles in infant EEGs has been limited to-date. In this study, we present a novel supervised machine learning-based algorithm to detect sleep spindles in infant EEG recordings, which has the potential to assist researchers and clinicians in the automated analysis of sleep spindles in infant EEG.
The conference was full of interesting talks and presentations, and it was my first time to attend a large virtual conference, which gave me a different experience.
NB: The paper is available here!
— Lan Wei
June Dublin Electrophysiology Research Clinic
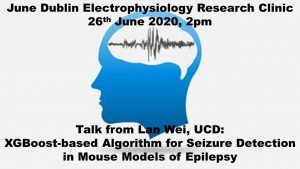
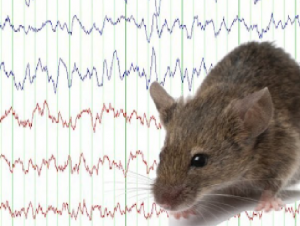
LiSDA @ ITiCSE 2020
We (Brett Becker and Catherine Mooney) are just “back” from the 25th Annual ACM Conference on Innovation and Technology in Computer Science Education (ITiCSE), an A-ranked conference that was held virtually on June 17 and 18.
We presented a poster entitled “Exploring Sense of Belonging in Computer Science Students” which won the best poster award. This poster, co-authored by UCD CS PhD students Anna Antoniadi and Ioannis Karvelas as well as UCD Physics PhD student Lana Salmon, explored the relationship between sense of belonging and participation in networking, outreach and mentoring activities.
Student sense of belonging has been shown to be associated with many attributes such as motivation and persistence. However, sense of belonging can show variations according to factors such as race and gender. In this study, we examined the relationship between undergraduate Computer Science students’ participation in networking, outreach, and mentoring activities and their sense of belonging. Results revealed lower levels of sense of belonging in women and self-identified minorities. However, we observed a higher sense of belonging in female students who participated in networking, outreach, and mentoring activities.
Overall these results will help us towards understanding what makes CS students feel included and what can be done to help those that don’t.
— Catherine Mooney
[pdf-embedder url=”https://lisda.ucd.ie/wp-content/uploads/2020/06/ITiCSE_2020_388.pdf”]
Manaz’s PhD Viva Voce
Huge congratulations to Manaz Kaleel who successfully defended his thesis at his PhD Viva Voce examination this morning.
His thesis title was “Improving Protein Structure and Subcellular Localization Prediction using Deep Learning” and the examiners were Prof Pier Luigi Martelli (University of Bologna) and Dr Vivek Nallur (UCD), and the chair was Assoc Professor Neil Hurley (UCD).
— Catherine Mooney
Yuhan Du’s Stage Transfer
Very excited to announce that Yuhan Du has passed her PhD stage transfer and progressed to Stage 2 of her PhD.
Great thanks from Yuhan to the LiSDA team for their help along the way. Everyone was very supportive and gave very helpful feedback. Couldn’t have done this without the team.
— Yuhan Du
ACM Announces New Members of Future of Computing Academy
The ACM Future of Computing Academy (FCA), created in 2016, brings together talented young professionals from a broad range of computing subdisciplines and institutions—academia, research labs, established companies, and startups—who want to perform valuable services for the community while expanding their professional networks and leadership experience. FCA members identify and implement pilot projects that address challenging issues facing our organization and the computing field in general. They work closely with ACM leadership, bringing in new ideas and perspectives and helping to integrate successful pilots—such as “crash courses” in AI and cross-disciplinary podcasts—into ACM’s institutional practices. FCA members are selected biennially through a highly competitive process similar to the nomination process for ACM awards.
Three women were selected to the FCA from Europe: Claudia Mazo, Leila Bahri, and Ella Peltonen.
Recognition of FCA members at the Grand Hyatt NY Hotel, New York.
We are wishing the best of luck to Dr Claudia Mazo in her career journey!
Teaching Machine Learning Courses in NUI Galway
Between 4/11/2019 and 8/11/2019, Dr Catherine Mooney was invited to teach an introduction to machine learning to the SFI Centre for Research Training in Genomics Data Science in NUI Galway. Yuhan Du and Lan Wei were invited to demonstrate for the lab sessions.
The courses were very informative and well-received. We were glad to know that the students learnt a lot about machine learning and how it could be applied to their research. It was a real pleasure to meet many staffs and students there. What a great week!
— Yuhan Du
ACM Celebration of Women in Computing WomENcourage 2019
Anna Markella Antoniadi, Lan Wei and Yuhan Du were all awarded scholarships to attend the ACM Celebration of Women in Computing WomENcourage 2019 in Rome, Italy.
They participated in the hackathon, presented their posters and met lots of great women from the field of Computer Science. The first day of the hackathon was long and tiring but a great experience and the first step to making new friends! The conference was full of interesting talks and presentations and Rome was just the loveliest place to be!
— Anna Markella Antoniadi
AIME 2019 in Poznan, Poland
I was thrilled to attend for the first time the Conference on Artificial Intelligence in Medicine (AIME) on June 26-29, 2019. I learned a lot at the Tutorial on Evaluation of Prediction Models in Medicine and the workshop on Transparent, Explainable and Affective AI in Medical Systems (TEAAM). And what a lovely group of scientists I met! I hope to see everyone again at AIME 2021 in Porto, Portugal!
P.S. You know you enjoyed it when you absolutely forgot to take pictures!
— Anna Markella Antoniadi
CS Sparks 2018
Exciting stuff happening at the UCD School of Computer Science! So happy to have the opportunity to help these girls get creative using code and Micro:bits! Winning the :MOVE mini buggies dancing competition was the cherry on top! Congratulations girls! It was indeed As Easy as pi.
— Anna Markella Antoniadi and Catherine Mooney