Lan attended the 2024 14th International Conference on Biomedical Engineering and Technology (ICBET 2024) which is held from June 14-17, 2024 in Seoul, South Korea. ICBET conference aims to bring together researchers, engineers, academicians, and industry professionals from around the world to present their latest research findings and advancements in the field of Biomedical Engineering and Technology.
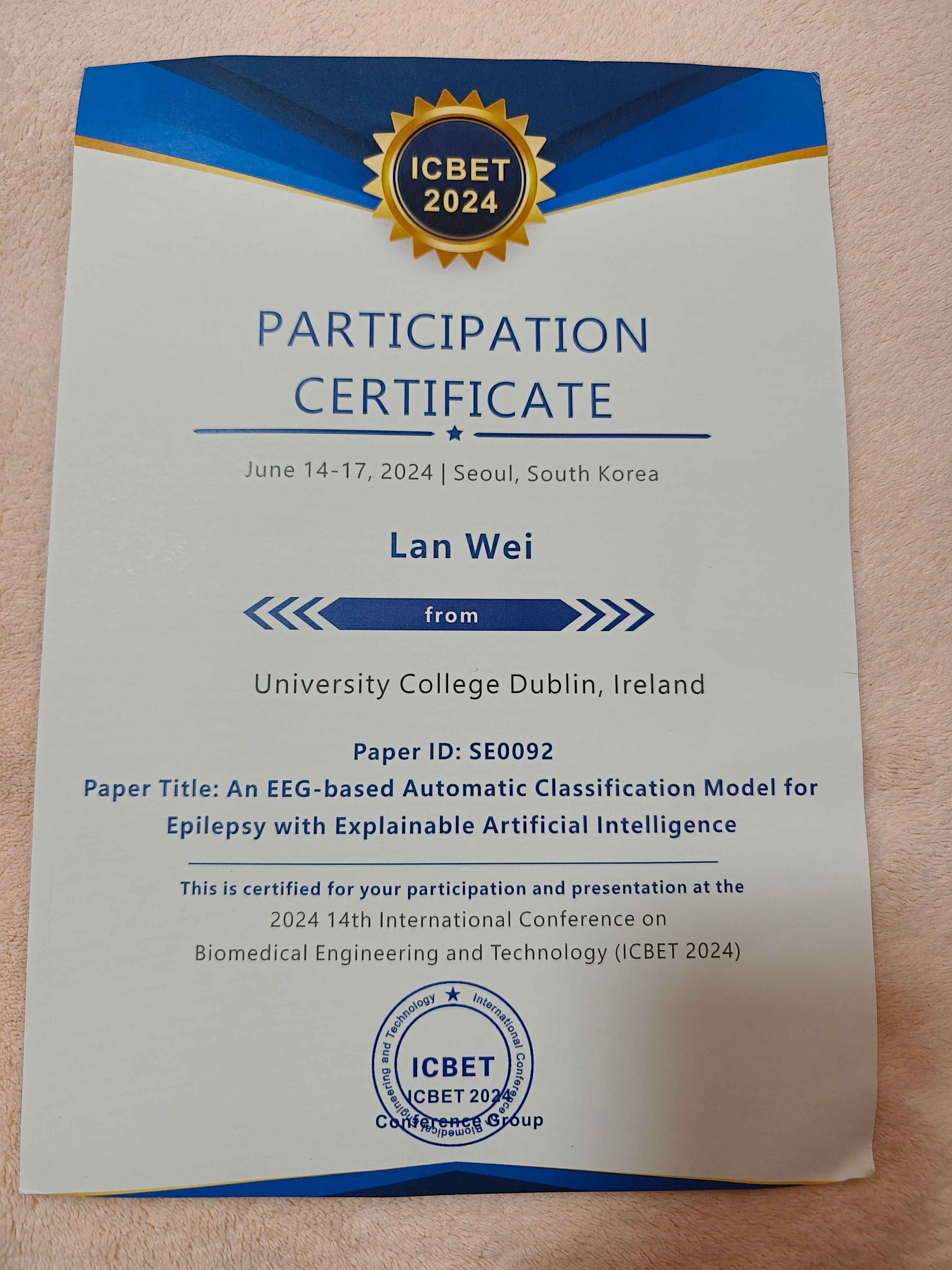
Lan presented their work co-authored by Dr. Catherine “An EEG-based Automatic Classification Model for Epilepsy with Explainable Artificial Intelligence”. Being part of the ICBET conference and engaging with fellow researchers in our field was an absolutely delightful experience!
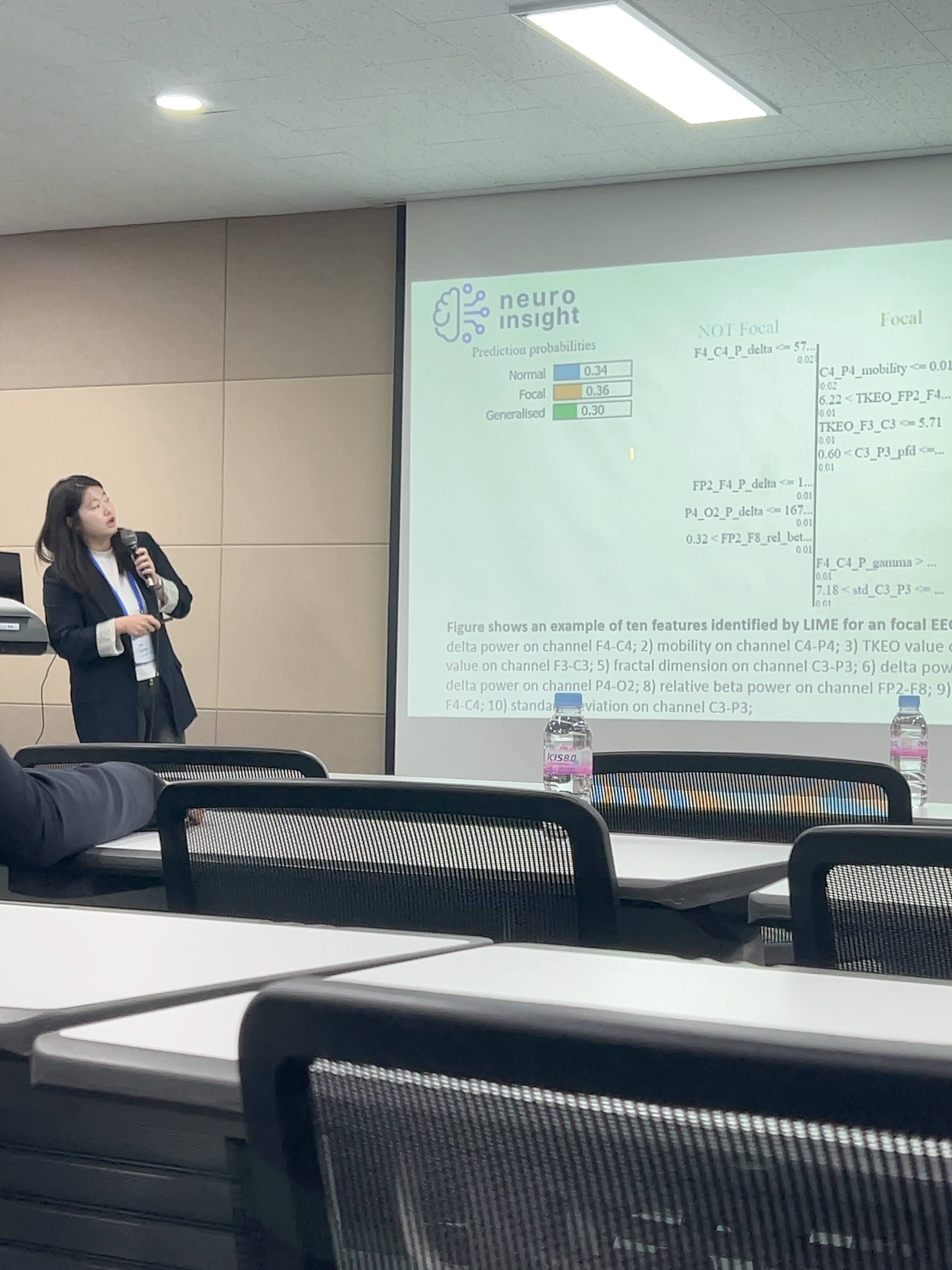
In addition, Lan was super happy to meet her best friend in Korea! After the conference sessions, Huiling and Lan set off on exciting adventures exploring Korea together!
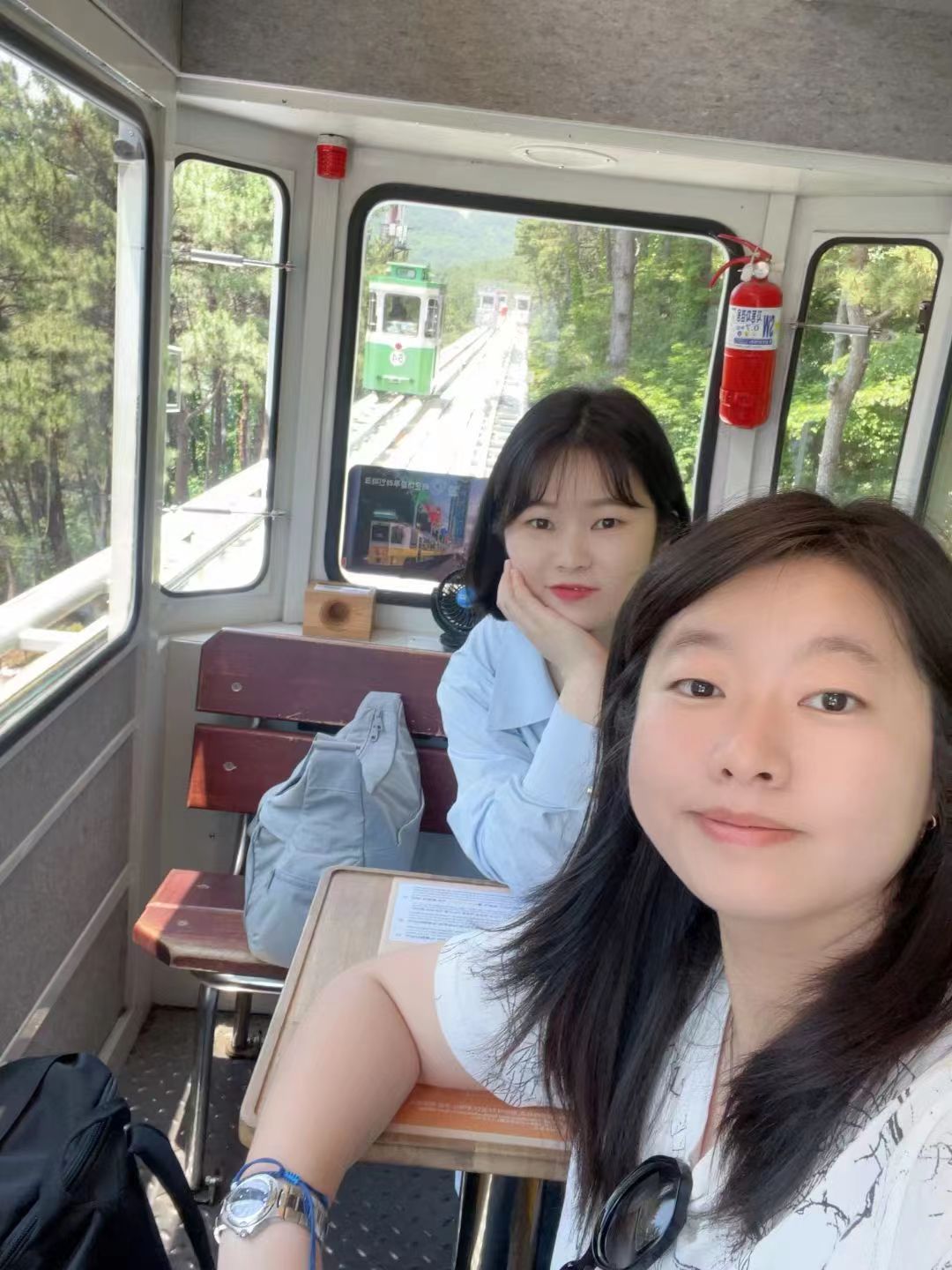
이번 한국 여행 정말 즐거웠습니다!
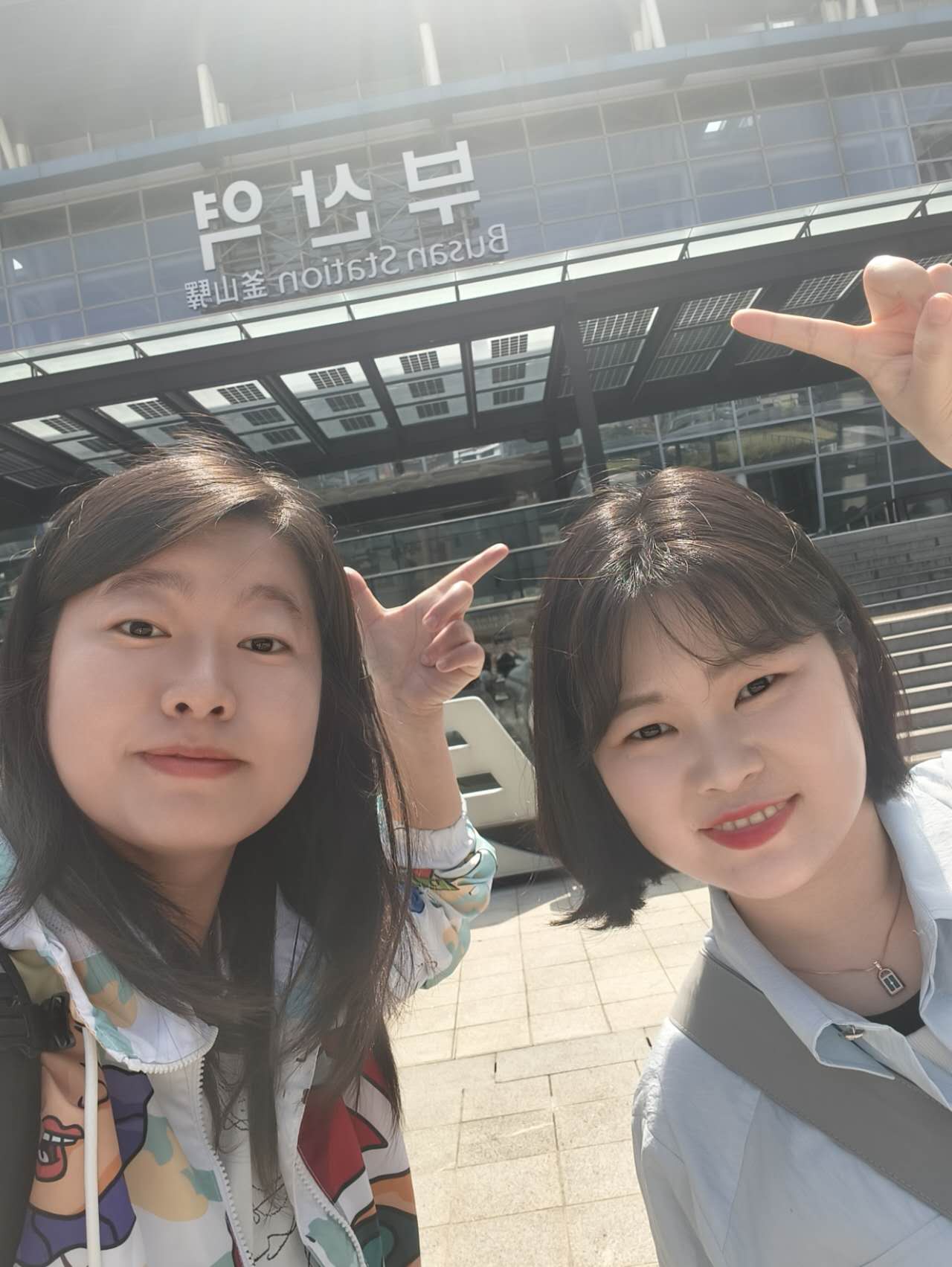
—–Lan